Using AI and satellite images to measure soil carbon
30 November 2022
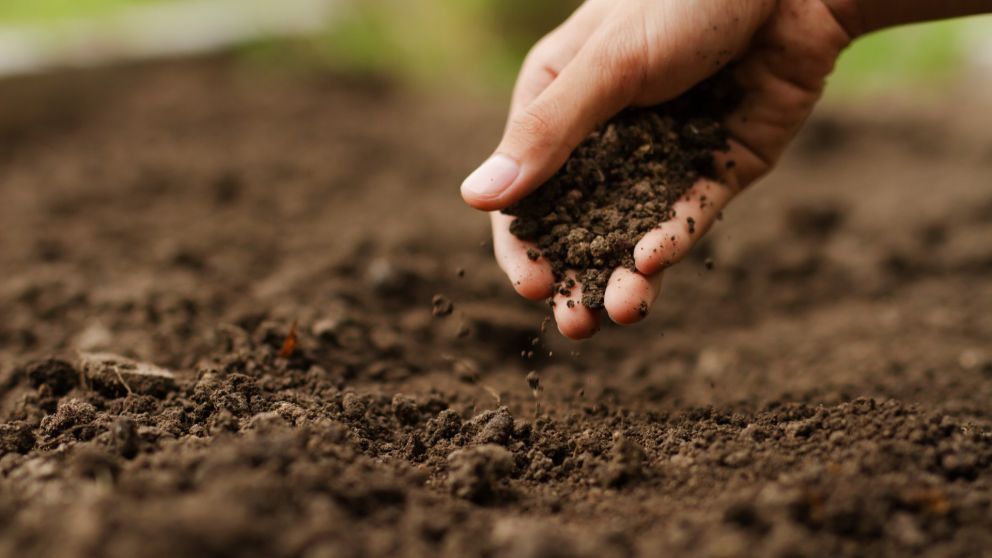
A project that uses artificial intelligence and satellite images to help determine the level of carbon content reliably in soil could be a game changer for farmers wanting to understand the carbon sequestration potential of their land, a Federation University researcher says.
Arif Rahman says the development of a cost-effective solution for determining carbon content in soils is appealing to farmers who are offered incentives to improve the content of their soil by adding carbon through fertilisers.
By using free images from NASA's Landsat and the European Space Agency Sentinel satellite, Mr Rahman's research will include the development of complex machine learning tools to provide an accurate estimation of carbon. The images will allow him to create 2D maps of farms and identify areas where farmers can prioritise carbon sequestration – the process of capturing and storing carbon.
The lack of affordable and practical carbon measurement methods has resulted in soil carbon sequestration being widely excluded from soil-based emissions abatement projects under carbon trading schemes.
"Carbon is generally good for soil and it's not the culprit in emissions – carbon dioxide is the concern, and scientists are working to capture carbon from carbon dioxide in the atmosphere and put it back into the soil," Mr Rahman said.
"In Australia, the Emissions Reduction Fund (ERF) provides incentives to farmers who adhere to carbon-friendly agricultural practices and capture more carbon into their soil, but traditional carbon measurement methods are expensive and invasive. Farmers need to send the soil samples to laboratories for testing, which is challenging for farmers who are often very time-poor.
"Using the maps and land coordinates, a body like the ERF could estimate the carbon without engaging the farmers."
Mr Rahman, whose study is co-sponsored by Soil CRC and the Wimmera CMA, says the benefits of capturing more carbon for farmers include being incentivised to do so while improving the quality of their land. On a global level, carbon sequestration contributes to the overall reduction of emissions and improves food security with better-quality soils.
For the project, Mr Rahman settled on hyperspectral images and evaluated whether the imaging could be done at ground level by farmers by using drones or satellite images. Scientists have long used hyperspectral images to effectively analyse the composition of materials like soils and chemicals by gathering information from across the electromagnetic spectrum.
"In terms of accuracy, closer proximity to the land results in more accurate results, and if you are on a farm, a hand-held hyperspectral camera will give slightly better results than a drone, but the accuracy levels are still very good. However, both are time-consuming and expensive," Mr Rahman said.
"With satellite images, the accuracy might be lower as the images are of lower resolution, but there are significant savings in cost and time."
A unique feature of the project will be the adoption of two separate models to improve accuracy – a physics-based model and a machine-learning model.
The physics-based method, more commonly known as a process-based method, typically involves estimating carbon content from an image using soil science expertise and formulating an equation. Different variables from the images and other sources are put into the equation – resulting in a calculation that may not be very accurate but offers an approximation.
"Physics-based methods works fine in simple mechanistic situations, but they do not incorporate real-world uncertainties," Mr Rahman said.
"Machine learning does not care about any predefined equations, it learns by examples, you are giving it photos and telling it what the carbon content is for particular images, and the model tries to learn the equation. The more examples you give it, the more accurate it will be.
"These two methods have been totally different disciplines and do not benefit from each other, but in my research, I'll be using machine learning methods, but my machine learning model will take inspiration from physics. This has been done successfully in other aspects of agriculture, like estimating soil moisture, but not for carbon."
Mr Rahman says a recent field trip to New South Wales to trial drone-based imaging demonstrated why satellite images could provide the perfect solution.
"From that experience, I saw that it was not a one-person job. It requires many people, and it also requires a lot of technical skills. Often, it's just one person or one family who manage hundreds of acres of farmland – they don't have time for this," Mr Rahman said.
"That's why participation in abatement programs is very low. My objective is to define a way to estimate soil carbon in a cost-effective and non-invasive way – but cost-effective mostly – so that more farmers are encouraged to participate."